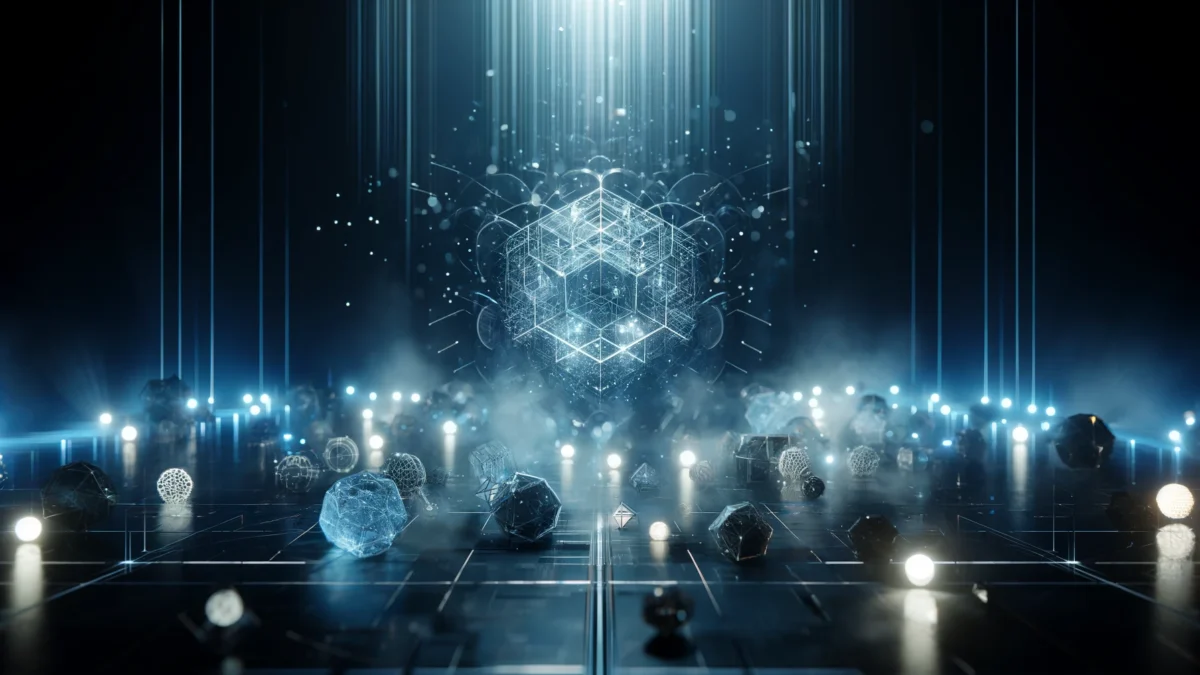
Cluster:
Building Digital Literacy
Citation:
Ranade N. (2024, April 15). Artificial Neural Networks and Human Biology: Going beyond computers to understand GPTs. Digital Life Institute. https://www.digitallife.org/artificial-neural-networks-and-human-biology-going-beyond-computers-to-understand-gpts/
Copy to Clipboard
As of early 2024, ChatGPT remains the first platform that garnered an estimated 100 million monthly users within just two months of launching in November 2022. While the record keeps growing – ChatGPT now boasts to have over 100 million weekly active users – criticism about the quality and accuracy of content produced by ChatGPT remains to be in question.
The early criticisms led to the popularity of the term AI hallucinations. An AI hallucination is defined as a process that occurs when an AI model generates inaccurate or misinformation but presents it as if it were a fact. Interestingly, in 2023, there was a 45% increase in dictionary.com’s lookups for “hallucinate” as compared to the previous year. This spike caught the attention of dictionary.com’s editors who chose it as their Word of the Year.
Although it became more popular since 2022, the first documented use of the word hallucination in computer science dates back to 1971 where researchers used it while discussing the quality of computers to accurately recognize handwriting. Due to the close association with the computer’s ability, or inability, to “see” (for recognizing human writing), the word hallucinate – derived from the Latin word ālūcinārī which means “to dream” or “to wander mentally” – was chosen. Similarly, many other terms for AI have been derived from those used to describe human behavior that is emulated by the computer, for example, making decisions, predicting or forecasting (the weather), assisting with tasks, and so on. The word ‘intelligence’ in Artificial Intelligence is also derived in the same way. It is used as a parallel to human beings’ intelligence which means “to understand”. The other, less popular reason for using ‘intelligence’ in the context of AI is due to the design of neural networks, a model used for machine learning, inspired by the neuronal organization found in the biological neural networks in human and animal brains.
A biological neural network is a network of neurons that are connected together by axons and dendrites that help organisms process information. It is an interconnection of processors or neurons that is found everywhere in an organism such as in the human brain, in an animal brain and in the heart, pancreas or lungs. The Artificial Neural Network (ANN) is inspired by the biological neural network. Because the connections are made up of hardware components that are artificially made (not naturally like the neurons), the term ‘artificial’ was used. ANNs are made up of layers of interconnected nodes, or neurons, that can process information and learn from data.
The human brain is a complex organ that is responsible for our ability to think, feel, and move. It is made up of billions of neurons that are connected in a complex network. These neurons communicate with each other by sending electrical signals. Here are some specific examples of how ANNs are inspired by human biology:
- Neurons: The basic unit of an ANN is the neuron, which is inspired by the biological neuron. Neurons in an ANN are simple processing units that receive input from other neurons and produce an output.
- Synapses: The connections between neurons in an ANN are called synapses. Synapses are inspired by the synapses in the human brain, which are the junctions between two neurons. Synapses in an ANN are responsible for transmitting signals between neurons.
- Learning: ANNs learn by adjusting the weights of the connections between their neurons. This process is inspired by the way that the human brain learns. When we learn new information, the connections between neurons in our brain are strengthened or weakened.
- Parallel processing: ANNs are able to process information in parallel, just like the human brain. This allows ANNs to solve complex problems quickly and efficiently.
Some other similarities are:
- ANNs are made up of layers of interconnected nodes, just like the human brain.
- ANNs use a similar learning algorithm to the human brain. This learning algorithm is called backpropagation by which ANNs learn from their mistakes. When an ANN makes a mistake, it adjusts the weights of the connections between its neurons. This process is repeated until the ANN learns to make fewer mistakes.
It should be no surprise that just like humans, ANN’s make mistakes and produce inaccurate information. However, the problem with any software generating misinformation is far greater than the same being done by humans. Bots used on social media in the 2016 elections demonstrate how computer programs can impact major events merely due to the volume of data they can generate in a short time. Aside from misleading people with factually inaccurate information and eroding user trust, when AI hallucinates, it can perpetuate biases or cause other harmful consequences for a huge number of people compared to that by a real human being. This is primarily because unlike humans, computer programs do not follow a default social order instilled through values such as integrity and credibility.
While ANNs mirror biology, regulations for human speech haven’t yet been mirrored for AI. There are three main challenges for AI oversight: dealing with the velocity of AI developments, parsing the components of what to regulate, and determining who regulates and how. Several countries have found their own regulation models. Most of these walk a tightrope between protecting the public interest and promoting innovation and investment. Does the key to regulation building also lie in emulating the socio-political systems developed by humans? That remains to be answered.